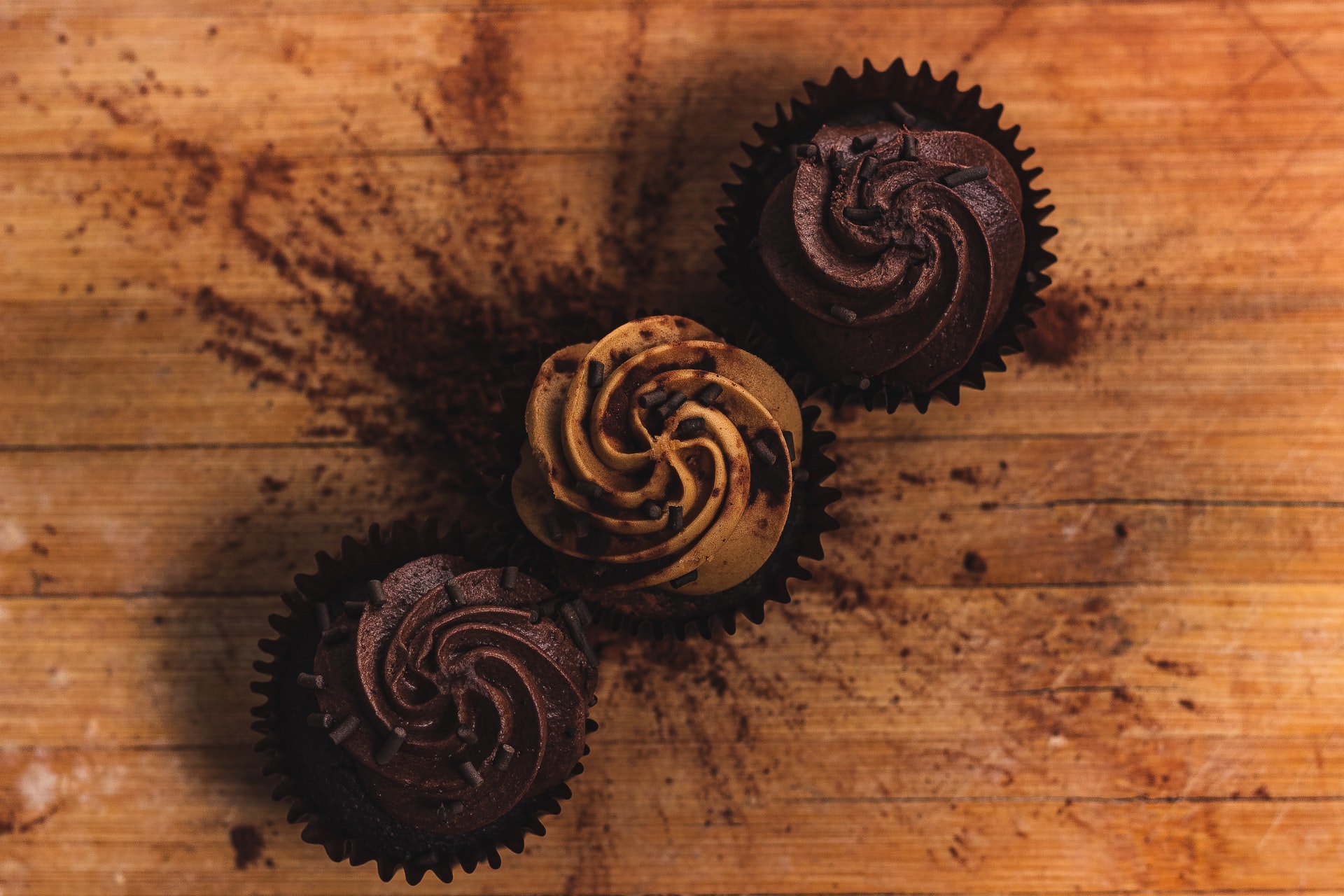
How can we use Data Science as a tool to address inequality?
We live in an increasingly data-driven world. But how can we use Data Science as a tool to address inequality? First of all, we can use it to generate knowledge, and understand what is going on in developing countries. To address a problem, you need to know what is going on. Second of all, closing the digital data gap is a step towards closing one form of inequality causing ineffective policy-making. We can use Data Science to create digital footprints of our population. And lastly, we can use Data Science as a tool for smart investment decisions in the private sector, converting it into a tool for enterprises to generate the most possible value out of their money.
Act 1: To address a problem, we need to know what is going on.
In 2006, Chile comes to the conclusion that an overproportional large share of its children younger than 4 years old is affected by poverty (CASEN (2006)). 10.5 percent of the overall population live below the poverty line, and 3.2 percent in extreme poverty, compared to 16.7 percent of children below 4 years old (7.2 percent respectively). At the same time, the National Survey of Quality of Life conducted in 2006 revealed that 30 percent of children below 5 years old did not reach their development goals, and that development delays mainly persisted in low income groups. These findings caused resonance in the political debate, and shortly thereafter, the Presidential Advisory Council for the Reform of Policies for Children was founded. Its purpose was to identify, formulate and design the establishment of a social protections system for Early Childhood in Chile, today known as Chile Crece Contigo.
This is one example showing how data helped to reveal a problem, then resulting in a targeted response. To make things better, we need to know what is going on. Another example is the High/Scope Perry Preschool Project. The High/Scope Perry Preschool Project started in 1962 analyzing the influences of pre-school education on children’s learning outcomes. The project started when David Weikert noted that poor children do much worse in school, and founded a committee trying to address this. As part of the project, a random group of vulnerable, ultra-poor children between 3 and 4 years old got access to pre-school as well as a weekly 90-minute home visits by a social worker, while a second group of vulnerable, ultra-poor children with similar characteristics served as their control group. 24 years later, researchers compared several socio-economic outcomes among both groups, as criminal activities, income, and educational outcomes. The relevations of the High/Scope Perry Preschool Project contributed to the general acceptance of the importance of kindergardens in society, and the foundation of a large number of pre-school facilities. Nowadays, the High/Scope Perry Preschool Project has been extensively studied, similar initiatives have been founded, and the knowledge created through data generated through these experiments flows into respective policy designs.
Act 2: Population Register - A digital footprint of a country’s population
So, if we need data to understand underlying problems, and to know how to then best address these, which form should our data have? Do surveys serve our purpose, or do we need to create a full picture of what is going on? The first known population census was conducted in 3800 BCE in The Babylonian Empire, then followed by China in 2 BCE (Source). The Incas had a census system based on knots on strings made from llama or alpaca hair, and the United States conducted its first census in 1790.
Recently, some countries have gone one step further, especially countries in Northern Europe, and created Population Registers, giving a regular, periodic full picture of its population. In these systems each resident residing in a country receives an electronic, anomymized identity number and remains in the system until they leave the country. Basic information tracked in the register is one’s personal data, as one’s name, address, citizenship, family relations, native language and birth of date. But the population register can also contain information about ownership. Norther-European countries have linked this data to additional registers, as for example linked employer-employee registers, containing detailed information about people’s work activity, wages, social assistance, as also information about enterprises.
So these countries have a pretty good idea of what is going on in their countries, and can therefore react fastly and swiftly to problems identified via their data. But there are still countries which have been without a census since 1990, as for example Afghanistan (1979), Eritrea (1984), Lebanon (1932), Somalia (1985), Uzbekistan (1989), and the area of Western Sahara (1970), putting serious restrictions on targeted policy-making. This is what one could call a “poverty data gap”. If Finnish statisticians can exactly identify in which neigborhoods investment in Water & Sanitation is needed, but statisticians in Afghanistan cannot even identify which share of their population has access to tab water, then this already creates a knowledge gap, and is only the beginning of a large rat tail.
Of course, under the ideal situation we would dispose about as much information as possible for effective policy design (securing data protection, and anonymization, of course), but data gathering is expensive. The OECD estimates that the total cost of a survey is approximately US-Dollar 100 per household in Africa and Latin America, US-Dollar 40-60 in East Asia and US-Dollar 25-40 in South Asia. So, sometimes we have to rely on samples, or small-scale samples. And recently, of course, Open Source Data.
Act 3: Limited resources and smart investment decisions
Act 1 and 2 have shown the underlying rational for how we can use Data Science as a tool for effective policy making to address inequality in the public sector. But what about the private sector? Is Data Science a valuable tool for firms and enterprises in developing countries to address the challenges they face?
Resources are limited, and companies have to make the best investment decisions possible with the limited resources they have at hand. This is where financial analyses, as well as economic analyses can help to shed some light. Let’s take Paraguay, for example. Paraguay is a land-logged country in the Southern Cone of Southamerica. Paraguay is the 4th largest exporter of soy beans in the World (Source) and recently surpassed Argentina for the eighth place among the world’s largest beef exports (Source). Let’s take an investor looking to invest in the forestry industry, for example. If we have a timber plantation with a financial rate of return in the North-West of the country of 10 percent, and a similar timber plantation resulting in a financial return of 12 percent in the South-East of the country, the investor prefers to move his money to the South-East, of course. Factors that drive the profitability of timber plantations are transport costs, average tree growth, soil adaptability, among others. So if we can plot a granular map of the average tree growth, or soil adaptability in Paraguay, we can help investors to make informed investment decisions, and generate the largest value possible with the same amount of money. But drawing this map is only possible if we dispose of the right data.
The ways in which Data Science can be transformed into a tool to address inequality are manyfold. I have only lifted out some of the possibilities in which Data Science can help us to make a contribution. Read my next blogpost about how to make the most out of Open Data Sources for development purposes.